The relative impact of marketing, research-and-development, and operations capabilities on firm performance
그동안 박사 자격시험을 준비하면서 정리한 내용을 하나씩 풀고 있습니다. 주로 유명한 마케팅 논문들이 업로드될 예정입니다. Marketing Strategy 중에서도 Role of Marketing에 해당하는 세 번째 논문 "핵심 요약"입니다.
- Abstract
- Marketing vs Research and development vs Operations capabilities → firm performance
- The impact of the marketing function on firm performance has been the focus of much recent research in marketing. Thus, the effect of marketing capability on firm performance, compared with that of other capabilities, such as research and development and operations, is an issue of importance to managers.
- Meta-analysis using a mixed-effects model
- To examine this issue and generate empirical generalizations, the authors conduct a meta-analysis of the firm capability–performance relationship using a mixed-effects model.
- Marketing capability ☆
- The results show that, in general, marketing capability has a stronger impact on firm performance than research-and-development and operations capabilities. The results provide guidelines for managers and generate directions for further research.
- Marketing vs Research and development vs Operations capabilities → firm performance
- Key — 마케팅 역량이 짱이다
- Takeaways
- RQ — marketing capability vs other capabilities on firm performance
- Whether marketing capability has a greater or lesser influence on firm performance than other capabilities
- What is the mean impact of capability on performance?
- Does the impact on performance vary for marketing, R&D, and operations capabilities?
- What other study characteristics moderate the overall relationship between capabilities and performance?
- Whether marketing capability has a greater or lesser influence on firm performance than other capabilities
- Definitions
- Marketing capability
소비자 니즈 파악, 효과적으로 offering 제공- A firm's ability
- to understand and forecast customer needs better than its competitors and
- to effectively link its offerings to customers (market sensing and customer-linking capabilities; Day 1994).
- A firm's ability
- Research-and-development capability
- firm's competency in developing and applying different technologies to produce effective new products and services
- Operations capability
자원을 최대한 활용하는 효과적인 공급자- the skills and knowledge that enable a firm to be efficient and flexible producers or service providers that use resources as fully as possible.
- Marketing capability
- Method
- Meta-analysis using a mixed-effects model
- Testing H1, H2, and the Impact of Study Characteristics Using a Mixed-Effects Model
- Mixed-effects model ⊃ HLMs (Hierarchical Linear Modeling)
- HLMs account for the nested structure of the data by modeling within- and between-study variances.
- The mixed-effects model we employed in this study assumes that the two types of variations in effect sizes can be explained by the type of capability and performance variables, as well as other study characteristics.
- Mixed-effects model ⊃ HLMs (Hierarchical Linear Modeling)
- Model
이렇게 2 층이어서 — Multilevel regression modeling이라 불리고, Level 1은 가설, Level 2가 nested문제를 해결하는 듯!- The relative impact of marketing, research-and-development, and operations capabilities on firm performance
- The relative impact of marketing, research-and-development, and operations capabilities on firm performance
- cf) Nested Data Structure & HLM
- Nested Data & HLM.pdf
- Summary
- Researchers often study how students’ academic outcomes are associated with the characteristics of their classrooms, schools, and districts. They also study subgroups of students such as English language learner students and students in special education. However, district personnel may not be aware that commonly used analytic methods might give inaccurate readings of the statistical significance of results when individual (student) data are nested within groups (classrooms, programs, schools) or when group-level data are disaggregated to predict individual outcomes. In these cases, it may be necessary to use multilevel regression modeling (also known as hierarchical linear modeling or linear mixed modeling) to analyze data.
- Multilevel regression modeling does not correct bias in the regression coefficient estimates compared with an OLS model; however, it produces unbiased estimates of the standard errors associated with the regression coefficients when the data are nested, and easily allows group characteristics to be included in models of individual outcomes (Snijders & Bosker, 1999; Raudenbush & Bryk, 2001; Bickel, 2007, Gelman & Hill, 2007; Hox, 2010).
- Definitions
- Multilevel regression modeling
- A set of statistical models that accounts for the statistical dependency that may result from nested data. When the assumptions of the models are met, they provide unbiased estimates of the standard errors associated with regression coefficients and allow group characteristics to be included in statistical models of individual outcomes (Snijders & Bosker, 1999; Raudenbush & Bryk, 2001; Bickel, 2007, Gelman & Hill, 2007; Hox, 2010). The approach is also known as hierarchical linear modeling or linear mixed modeling.
- Nested data
- When data are collected from multiple individuals in a group, the individual data are considered nested within that group.
- Multilevel regression modeling
- Why this primer?
- A common statistical method used to address this type of question is ordinary least squares (OLS) regression analysis. OLS regression, which can examine the strength and direction of the relationship between two variables while holding other variables constant, is used extensively as an exploratory, explanatory, and prediction tool. However, a standard OLS model may produce misleading results about the statistical significance of a relationship when it is used to analyze data collected from students in classrooms and schools because of this “nesting” of data—students nested within classrooms.
- A key assumption of OLS models (and several other common analysis procedures) is that each individual in the sample provides a unique piece of statistical information unrelated to the information provided by other individuals in the sample. Because students who attend the same school are likely to be more similar to each other than they are to individuals in other schools (they are drawn from the same communities, use similar school resources, have the same teachers), meeting this assumption can be difficult. A standard OLS regression can produce statistically unbiased estimates of the relationships among variables (regression coefficients); however, the nesting of students in schools leads to correlated observations (a dependency among the data) and the possibility of downwardly biased estimates of the standard errors associated with the regression coefficients. If adjustments are not made to the OLS model to account for the statistical dependency introduced by nesting, analysts can make substantive errors in interpreting the statistical significance of relationships (Raudenbush & Bryk, 2001).2
- Education researchers also frequently examine how group or organizational characteristics, such as the characteristics of schools, are associated with individual outcomes. Each individual in a group is assigned the same value for the group characteristic. However, a group characteristic that is disaggregated to the individual level cannot explain individual differences within a group. For example, the percentage of students who receive free or reducedprice lunches at a school cannot explain student-to-student differences in test performance within a school. A standard OLS model that includes a disaggregated variable will use the individual-level sample size, which is inaccurate for a variable measured at the group level, for determining statistical significance. This practice can also lead to incorrect conclusions about the significance of the relationships under investigation
- Testing H1, H2, and the Impact of Study Characteristics Using a Mixed-Effects Model
- Theoretical Framework for the Meta-Analysis
- Hierarchical Linear Modeling (HLM) and Controlling for the effect of other study characteristics
- cf) File-drawer problem
- We calculated availability bias-that is, the number of studies reporting null effects for the respective relationship that is necessary to reduce the cumulative effect to the point of nonsignificance-to allay concerns about the "file-drawer problem" (see Lipsey and Wilson 2001).
- Variance in the Capability-Performance Relationship: Test of Hypotheses and Study Characteristics
- Meta-analysis using a mixed-effects model
- Hypotheses
- H1: The capability-performance relationship is stronger for marketing capability than for R&D capability.
- H2: The capability-performance relationship is stronger for marketing capability than for operations capability.
- Result
- Marketing capability has a stronger influence on performance than R&D capability and operations capability.
- RQ — marketing capability vs other capabilities on firm performance
cf) 대학 & 대학원 입시 자기소개서를 "논문 연구"로 풍부하게 만들어 드립니다. 서비스가 궁금하다면?
https://beautifulresearch.tistory.com/39
대학, 대학원 입시 자소서 & 면접에 논문으로 깊이를 더해 드립니다! (입시, 면접, 대학자소서, 대
안녕하세요, KAIST 박사 Eager Beaver 입니다. "목표 학과에 열정"이 있다는 것을 드러내고 싶은데... 인터넷 검색으로 나오는 내용을 넣어보자니 출처도 애매하고.... 맞는 내용인지도 모르겠고.. 그렇
beautifulresearch.tistory.com
cf) 해외 논문 톱 저널 순서대로 신속하고 완벽하게 정리해 드립니다 (논문검색, 선행연구, 논문리서치, 사업근거마련) 서비스가 궁금하다면? https://beautifulresearch.tistory.com/40
해외 논문 톱 저널 순서대로 신속하고 완벽하게 정리! #선행논문 #논문검색 #논문리서치 #사업근
KAIST 박사 Eager Beaver입니다. 당신이 원하는 키워드의 논문, 톱 저널 순서대로 완벽 정리해 드립니다. [서비스 강점] 구글에서 하나하나 눌러 들어가도 보고, 스크롤도 내려도 보고.... 검색되는 논
beautifulresearch.tistory.com
— 해당 서비스를 이용하시면 Sci-Hub에 검색하여 다운받기에 용이하도록 DOI도 정리해드립니다
sci hub 참고: https://beautifulresearch.tistory.com/20
cf) 대학원생 스터디 톡방도 운영하고 있습니다 (계획, 기상 등 본인 원하는 것들 인증)! 연구에 외로운 대학원 동료분들 언제든 들어오세요! (https://open.kakao.com/o/gz2ZHI7c)
[글쓴이 소개]
서강대학교 수학&심리 복수전공 최우등 졸업
KAIST 마케팅 전공 석사 최우등 졸업, 박사과정 수료
자세한 소개는: https://beautifulresearch.tistory.com/7
'💟 심리학과 마케팅 이야기!' 카테고리의 다른 글
Business marketing 고객이 원하는 가치의 이해 (0) | 2021.09.07 |
---|---|
The impact of dynamic capabilities on operational marketing and technological capabilities (0) | 2021.09.07 |
회사의 구조적 특성, 전략적 강조점, 마케팅 행동이 핏이 맞아야 성과가 난다 (0) | 2021.09.07 |
대량 고객화 mass customization (0) | 2021.09.06 |
마케팅 근시안 Marketing Myopia? (0) | 2021.09.04 |
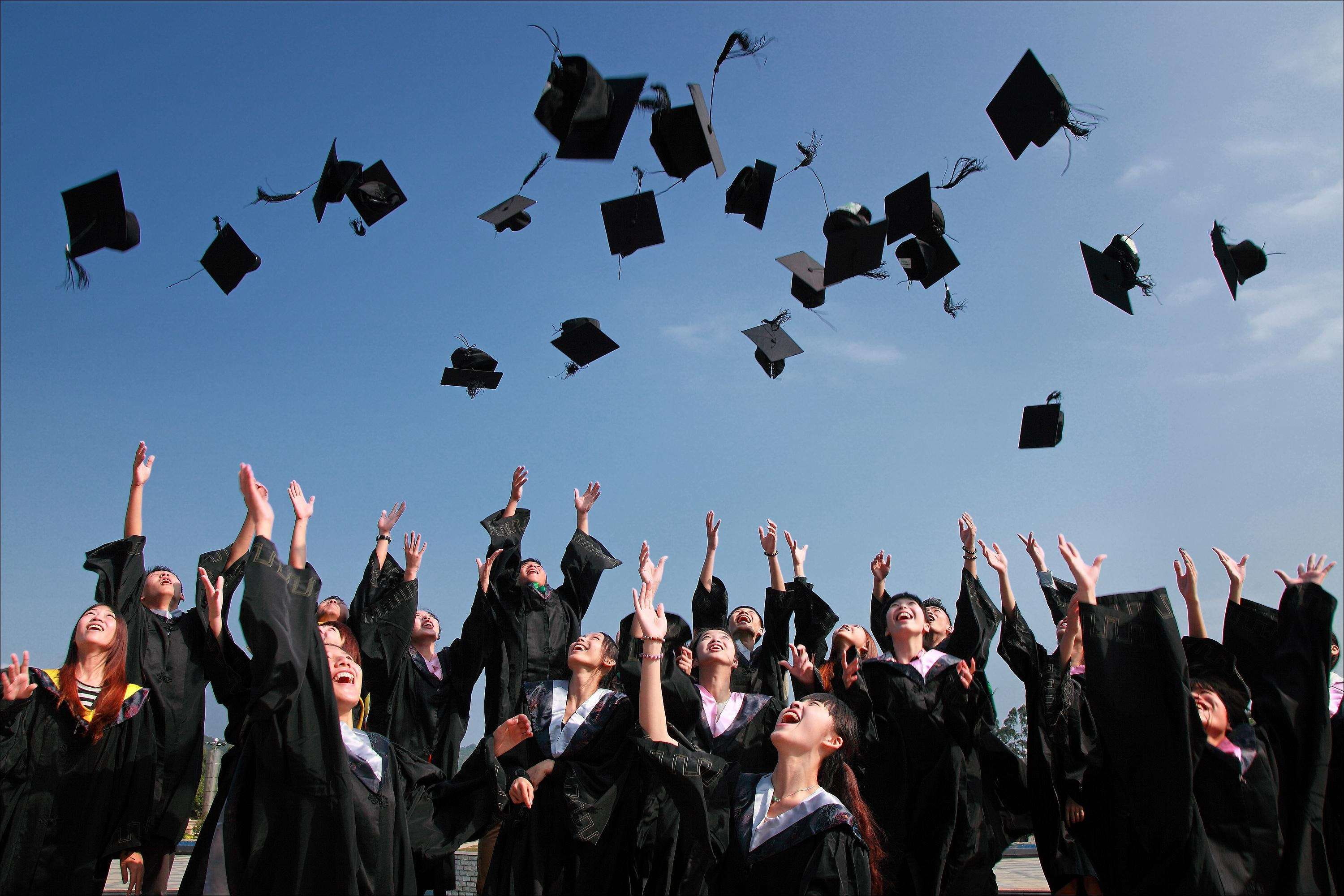
댓글